Navigating the AI Landscape: The Rise of Small Language Models Amidst the Dominance of Large Language Models
The Shift from Large Language Models to Small Language Models
Artificial intelligence has changed significantly in the last few years. This is especially true for relations between large and small language models. LLMs initially lead because of their superior abilities in text understanding and generation. Strong architectures support these abilities with nearly massive amounts of training data. The application of LLMs remains problematic and unaffordable. Their operational costs are high, especially due to the huge need for hardware and high energy consumption.
With the change in the AI landscape, organizations increasingly demand highly customized solutions that guarantee high efficiency without compromising performance. Such growing demand has placed SLMs more favorably in comparison with their bigger counterparts. Small language models are appreciated for having manageable footprints on resources that enable them to function well in standard hardware. That reflects a trend toward more practical applications of AI emphasizing more meaningful outcomes achieved by optimizing costs.
In niche applications, such as generative targeted content and conversational agents, SLMs are ahead; this further adds to their viability. To sum up SLM vs LLM, we assess the comparison between these models. The industry is shifting towards recognizing smaller models as capable of providing unique solutions. In this context, companies that have so far depended on large LLMs are now incorporating SLMs. They aim to ensure that the business model is an optimum balance. This balance considers the efficiency and capability of AI models. It also considers the needs and preferences of users.
A potential hybrid approach could fuse the strengths of LLMs and SLMs to create robust, efficient, and customizable solutions. This signals a readjustment within the AI world. It will also make sophisticated AI technologies more available to greater segments of users. Amidst mounting needs for sustainable, efficient models, SLMs are poised to take on an indispensable role in the future.
Benefits and Applications of Small Language Models
Small language models are rapidly being implemented within various industries. The key driving factor for this is the significant benefits that small language models have over bigger ones. One such benefit is that small language models are cost-effective. Organizations, especially the smaller ones with limited budgets, struggle to adopt LLMs. They cost much more in computations and multiple datasets. However, SLMs consume fewer resources and, therefore, organizations can capitalize on the powers of AI without stretching themselves too thin.
Another important feature of SLMs is efficiency. Smaller models usually have faster training times. They also have faster inference rates. This concept translates directly to practical use in real-time settings where rapid responses are paramount. For instance, customer service on platforms utilizes SLMs. Instant response to user queries enhances the user’s experience and satisfaction. Another aspect of this efficiency is that it supports iteration. It also meets specific compliance or needs in different industries.
The performance of SLMs represents the differentiation in the AI horizon. LLMs have better language understanding due to their large training. They can fine-tune for a particular application and thus improve its relevance and effectiveness. For example, in healthcare, SLMs may be trained on information about patients. They provide suggestions in diagnostics with more accurate insights based on patient characteristics. Additionally, the finance industry can use SLMs in fraud detection. They are also useful in risk assessment. This makes them handy for targeted applications.
With the increasing recognition of these attributes, the future of AI models for SLMs is bright. Balancing cost, efficiency, and tailored performance is crucial. Understanding the possibility of these models as a viable alternative to large language models is possible. The choice will depend on specific business needs. However, SLMs undoubtedly provide very compelling solutions across a wide range of applications.
Performance Comparison: Small Language Models vs. Large Language Models
The performance differences between small language models and large language models are crucial. They constitute a critical subject matter of debate in the current scenario of artificial intelligence. LLMs are widely publicized for their extensive capabilities. They demonstrate high accuracy on complex tasks. SLMs have received significant recognition for specific advantages within their specific environments. Performance is different about accuracy, efficiency, resource requirements, and overall suitability for enterprise applications.
Large-scale LLMs are generally better at generating nearly indistinguishable human responses. They excel in answering complex questions. However, these capabilities come at a cost of increased resource intensiveness. Such gigantic models require a lot of memory and computational power. This means that it might be challenging for smaller organizations. They often face difficulties if they lack appropriate infrastructure. Furthermore, querying LLMs always leads to latency, where such applications require immediate returns.
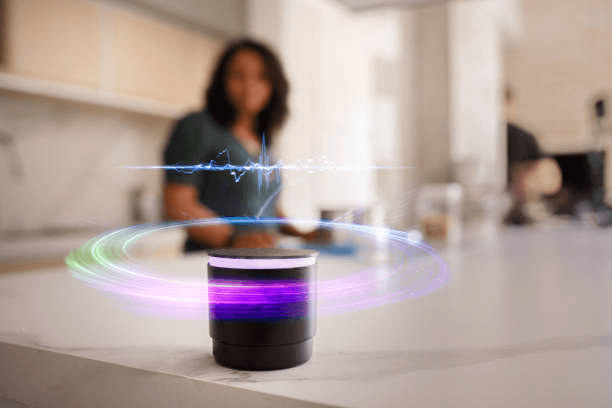
SLMs are more resource-effective. They are better in environments where both speed and computational budget are essential. They can be best suited for simpler or more narrow tasks where the complexity LLMs offer is predominantly deemed unnecessary. For example, SLMs can be suitable for customer service automation. They exhibit satisfactory performance in chatbot functionalities while being less resource-intensive.
Also, the rise towards SLM marks some continued trends within the AI model development. As companies face the real challenges and shortcomings of LLMs, they deal with costs and operational inefficiencies. It seems SLMs may be a practical alternative in ushering in innovation. They also help in adapting usage. Therefore, the future seems to favor both categories of AI models. The AI models are balanced toward strengths that align with an enterprise’s current needs.
Future Trends in AI: The Growing Importance of Specialized AI Models
As the AI landscape is now evolving, more focus is being given to developing special models of AI-SLMs. These are coming out as pretty strong alternatives for LLMs, particularly due to their efficient performance in multiple applications. Targeted functionalities: SLM involves targeted functionalities. They aim to enhance the efficiency of AI models. In particular, their functionality focuses on providing specific tasks. These tasks may benefit a business seeking certain AI solutions.
SLMs in AI: Specialization for Cost-Effective Solutions and Competitive Advantage
The trend of developing SLMs is on the rise. This indicates specialization in the domain of AI. Businesses are becoming more aware of how often one-size-fits-all solutions fall short of their unique needs. That means less data can be used to fine-tune SLMs. This approach makes it cost-effective and reachable for smaller enterprises. These enterprises may not possess a broad resource. This is essential because more adaptability requires competition in allowing firms to become competitive through AI usage in operations.
Furthermore, with the growing power of SLM, substantial implications can be drawn for disciplines such as healthcare, finance, and marketing. For instance, in health care, small language models can scan patient data. They provide interpretations in a non-public way and with privacy compliance. In this respect, focused models not only make the process easier but also increase sharpness in prediction. Case studies of companies using SLMs show significant gains in efficiency and effectiveness for bottom lines. That is, smaller models can compete with large, lumbering language models as targeted models.
Small language models will probably be the center of it all. Their benefits will perhaps become the driving force for novel applications and solutions. These advances could form a new standard in the artificial intelligence development arena. Moving from LLMs to SLMs may empower businesses. They can leverage AI in areas that best solve the particular challenges they face in their specific domains.