Accelerating Breakthroughs: The Role of AI in Scientific Discovery
The role of Artificial Intelligence (AI) is revolutionizing scientific research by enhancing data analysis, modeling, and discovery processes across various fields. AI increases the speed of innovation. It automates complex tasks and provides predictive insights. This enables researchers to tackle pressing global challenges more effectively. This blog examines how AI tools are changing the landscape of scientific inquiry. They are driving transformative breakthroughs in health and environmental science, and beyond.
Introduction to the Role of AI in Scientific Research
The role of AI has become a pivotal force in scientific research. It reshapes methodologies and enhances the efficiency of discovery processes across various disciplines. The concept of AI dates back to the mid-20th century. Initially, efforts focused on developing algorithms capable of mimicking human cognition. Since then, computing power has advanced significantly. Algorithm design has also improved. These developments have facilitated the evolution of AI. AI can now tackle increasingly complex problems.
As we progress through the 21st century, the integration of AI into scientific research is becoming more widespread. This integration is not limited to computational fields; rather, it spans areas such as biology, chemistry, physics, and environmental science. AI technologies help researchers analyze vast datasets more efficiently. They reveal patterns and insights that would be impractical to uncover through traditional methods. For instance, machine learning algorithms can identify relationships within genetic data, facilitating breakthroughs in personalized medicine and genomics.
The Role Of AI in Scientific Discovery: Accelerating Research and Innovation Across Fields
The transformative potential of AI in scientific discovery is evident in several landmark achievements. AI-driven simulations and predictive models are streamlining experimental design, thereby reducing the time and resources required for research. In fields like materials science, AI has been instrumental in identifying new materials with desired properties. This significantly speeds up the development process. Furthermore, natural language processing capabilities allow researchers to sift through extensive scientific literature. They extract relevant information and accelerate their research endeavors.
In summary, the role of AI in scientific research is multifaceted and evolving. As advancements continue, AI is ready to revolutionize the way scientists work. It fosters unprecedented collaboration and innovation in discovery processes. This ongoing emergence promises to enhance efficiency. It also aims to catalyze breakthroughs that could redefine the boundaries of scientific knowledge.
Benefits of AI for Scientists
The integration of artificial intelligence (AI) within the scientific community has led to a significant transformation. It offers numerous advantages. These advantages are reshaping the landscape of research and discovery. One of the primary benefits of AI is its ability to dramatically enhance efficiency in data processing. In fields such as genomics and climate science, vast amounts of data are generated. Traditional methods of analysis can be exceedingly time-consuming. They are also prone to human error. AI algorithms can process large datasets quickly. They analyze and interpret information at speeds far surpassing human capability. This allows scientists to derive insights quickly and accurately.
Moreover, AI improves predictive capabilities, which are essential for hypothesis testing and forecasting in scientific research. By employing machine learning models, researchers can identify patterns and make predictions based on historical data. For instance, in drug discovery, AI predicts the effectiveness of various compounds. It significantly reduces the time required to identify viable candidates for further testing. This predictive power can also facilitate the understanding of complex systems. It ranges from predicting climate change effects to modeling biological processes. This fosters informed decision-making based on reliable projections.
The Role Of AI in Research: Streamlining Workflows and Accelerating Scientific Breakthroughs
Additionally, AI tools can streamline workflows, allowing scientists to focus on high-level inquiry rather than mundane tasks. Automated systems powered by AI can handle routine laboratory processes. They also manage data collection and even preliminary analyses. This frees researchers to devote more time to exploring innovative ideas and complex problems. A poignant example is in astrophysics. AI has enhanced the efficiency of analyzing astronomical data. It helps identify new celestial objects and accelerates discoveries in this vast field.
In summary, the myriad benefits of AI for scientists include improved efficiency in data processing. It also offers enhanced predictive capabilities and streamlined workflows. These benefits contribute to advancing scientific discovery across various disciplines. By leveraging AI, scientists can pursue deeper inquiries and foster breakthroughs that would otherwise be unattainable.
Accelerating Research with AI
The infusion of artificial intelligence (AI) into the scientific research domain is changing how discoveries are made. This change enhances the speed and efficiency of the research pipeline. AI uses machine learning and data analysis. These tools have begun to streamline various stages of scientific inquiry. The process extends from hypothesis generation to experimental design. This capability is increasingly vital in disciplines ranging from biology and chemistry to materials science and physics.
One notable example of AI’s impact is its application in drug discovery. Traditional methods for identifying potential drug candidates can be painstakingly slow and resource-intensive. However, AI algorithms can analyze vast datasets. They identify patterns and predict molecular behavior. This significantly expedites the identification of lead compounds. In recent projects, AI has enabled researchers to significantly reduce the time for the initial screening of drug compounds. The time has been cut from years to mere months if not weeks.
The Role Of AI in Experimental Design: Optimizing Research and Advancing Synthetic Biology
Another critical phase where AI enhances research is in experimental design. By employing algorithms that can model complex systems, scientists can optimize their experimental conditions before conducting physical trials. This predictive capability saves time. It also conserves resources. This allows researchers to focus on the most promising avenues of investigation. A case in point is the work conducted in synthetic biology. In this field, AI tools engineer microorganisms. These microorganisms are capable of producing biofuels more efficiently than traditional methods.
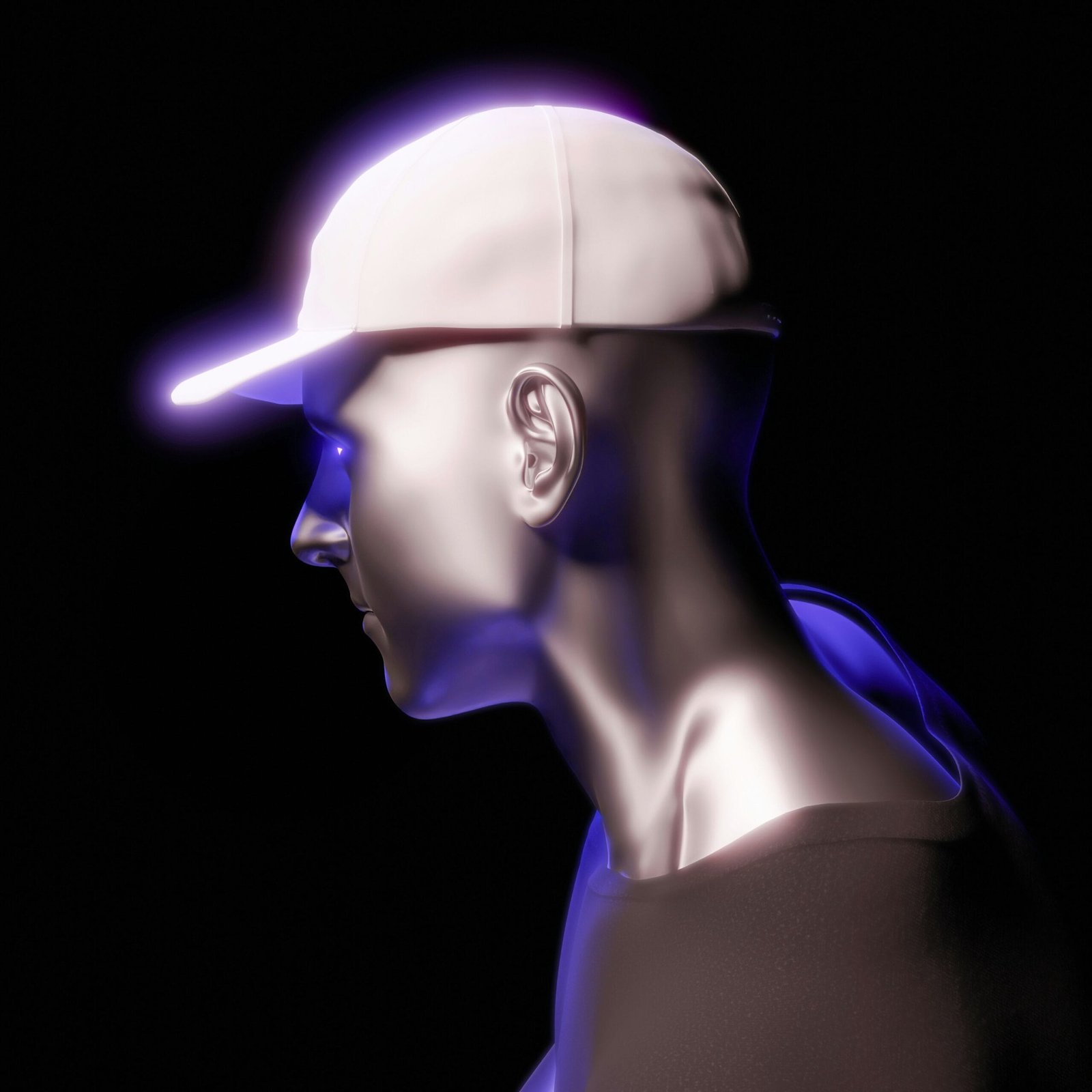
Further, AI has proven invaluable in data interpretation. Advanced machine learning algorithms can process and analyze large datasets with a speed and accuracy unattainable by human researchers. In fields such as genomics, AI assists scientists in uncovering genetic markers associated with diseases. This can lead to breakthroughs in personalized medicine. As a result, the role of AI in scientific research is not merely supplementary. It is foundational in driving rapid advancements and fostering innovation.
AI Tools for Data Analysis
AI has profoundly transformed the landscape of data analysis in scientific research. It offers tools that streamline the processing of large datasets. These tools reveal hidden patterns and produce actionable insights in real time. One of the most notable AI tools is TensorFlow, an open-source platform developed by Google. It enables researchers to implement complex machine learning algorithms and has become a go-to framework for running deep learning models. Its extensive library of pre-trained models allows for quick adaptation to varied scientific inquiries, from genomics to climate modeling.
Another significant player in the AI data analysis arena is IBM’s Watson. Known for its natural language processing capabilities, Watson helps researchers sift through vast volumes of literature and extract pertinent information. This capability aids scientists in formulating hypotheses and interpreting results efficiently. Watson is acknowledged for its ground-breaking applications in fields like healthcare. It facilitates the extraction of crucial insights from patient data. This enhances predictive analytics.
AI-Powered Data Analysis: Tools Like RapidMiner and KNIME Transform Scientific Discovery
Data analysis for scientific discovery has also benefited from specialized software. Examples include RapidMiner and KNIME. Both provide user-friendly interfaces that allow non-programmers to build analytical workflows while harnessing the power of AI. RapidMiner excels in predictive analytics. It enables researchers to build models that forecast outcomes based on historical data. KNIME is remarkable for its versatility in data integration and machine learning implementations.
Furthermore, methodologies such as reinforcement learning and unsupervised learning have garnered attention for their efficiency in optimizing large datasets. These techniques not only facilitate the identification of patterns but also enhance the decision-making process in research. The capabilities of AI continue to expand. Its role in revolutionizing data analysis also grows. This development ultimately accelerates the pace of scientific discovery across various disciplines.
Machine Learning in Discovery
Machine learning (ML) has emerged as a pivotal tool in scientific discovery. It enables researchers to analyze vast datasets with unprecedented efficiency. At its core, machine learning involves algorithms that learn from and make predictions based on data. These algorithms can be broadly categorized into supervised, unsupervised, and reinforcement learning methods. Supervised learning relies on labeled datasets to train models, while unsupervised learning uncovers hidden patterns in unlabeled data. Reinforcement learning, on the other hand, involves agents who learn to make decisions by receiving feedback from their environment.
One of the most significant applications of machine learning is in genomics, where it has revolutionized the analysis of genetic data. For instance, researchers have utilized ML algorithms to identify genes associated with particular diseases by analyzing complex genomics sequences. In a notable case, the integration of ML techniques has led to the identification of biomarkers that enhance the understanding of cancer progression, providing pathways for targeted therapies.
The Role Of AI in Drug Discovery: Leveraging Machine Learning to Accelerate and Cost-Optimize Research
Similarly, in drug discovery, machine learning methods are streamlining the identification of potential drug candidates. By applying algorithms to molecular data, researchers can predict which compounds are most likely to interact effectively with target proteins. An exemplary case is the use of deep learning, a subset of machine learning, to predict drug interactions quickly and accurately. This not only accelerates the drug development process but also reduces costs significantly.
Moreover, the field of materials science has greatly benefited from machine learning through the discovery of novel materials. Advanced algorithms can predict the properties of materials before they are synthesized in the laboratory. This approach has led to breakthroughs in manufacturing processes and the development of high-performance materials, illustrating the profound impact of machine learning on scientific research.
Future of AI in Research
The future of artificial intelligence (AI) in scientific research holds immense potential, promising to revolutionize methodologies and broaden the horizons of discovery. As emerging technologies evolve, AI is expected to play a critical role across various disciplines, enhancing the capacity for data analysis and interpretation. For instance, machine learning algorithms are increasingly being utilized to identify patterns in vast datasets that were previously unattainable through traditional methods. This capability could significantly shorten research timelines and facilitate more accurate predictions, which is particularly beneficial in fields like genomics and drug discovery.
Additionally, natural language processing (NLP) technologies are anticipated to transform how researchers conduct literature reviews and synthesize information. With NLP, AI tools can parse through extensive academic publications, extracting relevant findings and identifying knowledge gaps that may otherwise go unnoticed. This has the potential not only to improve the efficiency of literature reviews but also to inspire new research avenues based on the synthesized data.
AI-Driven Collaboration: Fostering Interdisciplinary Innovation in Scientific Research
Experts in the field highlight that the integration of AI into research practices may also lead to more collaborative and interdisciplinary efforts. For example, AI-driven platforms can facilitate collaborations among scientists from various domains, enabling diverse perspectives to converge on complex problems. This collaborative approach could drive innovation, resulting in breakthroughs that would be difficult to achieve in isolated research environments.
Furthermore, ethical considerations and transparency in AI applications will be crucial as the technology advances. Addressing these issues will help build trust among the research community and the public while ensuring that the benefits of AI are maximized responsibly. Overall, the horizon for AI in scientific research is expansive, with the potential to reshape our understanding and exploration of the natural world.
Ethical Considerations of AI in Science
The integration of artificial intelligence (AI) in scientific research brings forth substantial ethical considerations that warrant scrutiny. As AI technologies become increasingly involved in data analysis and experimental design, issues regarding data privacy, algorithmic bias, and accountability emerge as central topics of discussion. The handling of sensitive data, particularly in fields such as biomedical research, necessitates stringent measures to protect individual privacy. Researchers must ensure that data collection methods adhere to established ethical standards, ensuring that participants are informed and consenting to how their information will be used.
Moreover, the potential for bias in AI algorithms presents a significant challenge. Machine learning models are trained using historical data, which may contain pre-existing biases inherent to the datasets themselves. If not addressed, these biases can perpetuate systemic inequalities, skewing research outcomes and impacting decision-making processes. For instance, insufficient representation of diverse populations in medical research could lead to AI systems that do not effectively serve all demographic groups equally. As such, it is vital for scientists to rigorously evaluate their algorithms and the datasets used for training, ensuring that diversity and inclusivity are integral to their development.
Accountability and Ethics in AI-Driven Research: Ensuring Responsible and Transparent Practices
Accountability for AI-driven research further complicates the ethical landscape. When AI systems produce results that guide scientific inquiry, the question arises: Who is responsible for errors or adverse outcomes? Establishing a clear framework for accountability is essential to mitigate risks associated with AI usage in scientific endeavors. Regulatory frameworks are equally important; they could provide guidelines for the ethical deployment of AI technologies within research contexts. Such regulations would not only promote responsible use of AI but also cultivate public trust in scientific processes. In conclusion, addressing these ethical concerns is crucial for fostering a research environment that responsibly harnesses the capabilities of AI, while upholding the principles of integrity and respect in the scientific community.